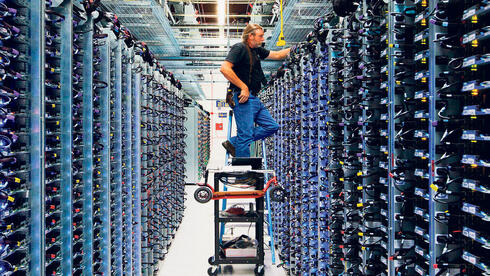
Size doesn’t matter: Bigger isn't always better in AI
Size doesn’t matter: Bigger isn't always better in AI
With exposure to ChatGPT, we were introduced to a new concept: a large language model, the "engine" behind the chatbot, which trains on hundreds of billions of data items. Now, the time has come for smaller and cheaper models, which can both perform well and quickly, giving smaller players a competitive edge.
When OpenAI's ChatGPT was revealed to the public in the fall of 2022, igniting the generative artificial intelligence (GenAI) revolution, it introduced us to the concept of a large language model (LLM). An LLM is an AI model with tens or hundreds of billions of parameters, which enables tools to answer questions, conduct conversations, analyze documents, create prose, and more, thanks to its extensive access to a significant portion of human knowledge and its innovative capabilities.
This revelation also sparked an arms race among AI companies, particularly technology giants, to develop the largest and best LLMs. This race is very expensive. The development of GPT-4, OpenAI's most advanced LLM with more than a trillion parameters, cost over $100 million. Development requires access to costly computing and processing resources, which have become more expensive and scarce due to the global competition. Operating these models is also expensive in terms of processing power, energy consumption, and overall cost.
As a result, the field has largely been dominated by companies that can afford these expenses, such as technology giants like Microsoft, Google, and Meta. However, a new trend is emerging: the development of not only large and expensive models but also smaller models, called MLMs (medium language models) and SMLs (small language models). These smaller models can have fewer than 10 billion parameters, cost up to $10 million to train, and require fewer resources.
Sometimes tailored to specific tasks, these smaller models can provide better or more efficient results under certain conditions compared to their larger counterparts. They represent the next step in the GenAI world, potentially increasing competition by opening opportunities for startups and companies with more limited resources.
Local processing without the cloud
"The topic of small and focused models is gaining momentum in the market, and we see it," Ori Goshen, co-founder and CEO of AI21 Labs, a leading Israeli company in the GenAI field, told Calcalist. "The reason is that we end up implementing these models within applications, and when the models are smaller, the cost is lower, and they are faster. This is a key consideration for those who build applications, whether corporate or consumer. Everyone who develops an application reaches a stage where they look at costs and response times because this affects the user experience and usability."
This trend is being led by the same companies that launched the current revolution. Microsoft, which invested $13 billion in OpenAI, highlights its small family of models, known as Phi. CEO Satya Nadella says these models have only a fraction of the parameters of the model behind the free version of ChatGPT but perform many tasks almost as well.
One of the advantages of small models is their ability to run on devices locally, without sending information to the cloud and relying on expensive data centers. The AI computers from the Copilot+ series that Microsoft introduced in May, expected to arrive from all major PC manufacturers in the coming weeks or months, include models that can perform actions such as producing images and answering queries locally.
Apple, the new player among the giants in this field, also plans to use small models that will leverage the company's powerful processors to answer questions quickly, securely, and privately. Only in cases where the small model cannot provide an appropriate answer will information be sent to the cloud for the use of larger models. Google and leading startups such as Anthropic, Mistral, and Cohere also launched small models this year. Even OpenAI, the founder of the large model genre, launched a smaller, cheaper-to-operate version of GPT-4 and plans to roll out smaller models in the future.
"You don't need a quadrillion operations to calculate how much 2 plus 2 is," said Ilya Polosukhin, founder of the blockchain company NEAR Protocol. In his previous position at Google, he was among the authors of the groundbreaking academic paper, "Attention is All You Need," which laid the foundations for generative artificial intelligence. This paper describes an architecture for building AI models called Transformer, whose implementation enabled the creation of LLMs. The transition to smaller models relies, in part, on a new architecture called Mamba, developed about six months ago by researchers from Carnegie Mellon and Princeton Universities.
"The good news of Mamba is the ability of a model to have a longer context (the input fed to the model in a query) without losing efficiency," Goshen told Calcalist. "In Transformer, when you increase the context, efficiency decreases, and it is very expensive to handle a lot of information. Mamba is a very efficient architecture, but there are tasks where the quality is less good. It's a trade-off. Not long ago, we released a model called Jamba, a relatively small model that combines the two architectures. We managed to produce a model that benefits from both worlds, efficiency and quality. Today it is already in its second version and can handle long contexts accurately.
"This is related to small models in that if you have a large model in terms of parameters and with a large context, you need a lot of hardware to run it. It is very expensive. We see beyond that some tasks can be solved using smaller models, and with Mamba, a small model in terms of parameters but with a relatively large context can receive a lot of information."
These small models can, for example, be dedicated models for professional use, such as a model trained on legal judgments and court protocols intended for jurists and lawyers. These models will use fewer parameters but will need to handle longer input because questions from professionals in their fields of expertise are often more complex and detailed than those from the general public. However, these models are not required to perform general actions and answer questions from diverse fields, so the number of parameters used for their training will be significantly smaller.
Specific tasks
Small models can also be general language models and answer diverse queries. "A small model can be a general model for simple queries; it depends on the complexity of the query," Goshen explained. "When you ask questions about a document, the question can be complex, and then a small model will not be enough to produce an accurate answer. In the future, we will see a combination of small and large models. We will be able to handle some things in a relatively efficient way, and for some, it will be worth paying more to receive treatment done by a more powerful model. There will also be small models that know how to handle certain tasks. For example, a small model that is good for a certain type of document. This is useful for an organization that has a lot of data and needs to perform a specific task, achieving even better results this way than with a large model. The combination of a small model with a large context is powerful."
Some organizations are already doing this. According to the Wall Street Journal, the credit rating company Experian switched from using large models to small models to operate the chatbots it uses to provide financial advice and customer service. According to the company's VP of data, Ali Khan, after being trained on the company's internal information, the models provided results as good as those of the large models but at a fraction of their cost.
The small models are also expected to revive competition in the GenAI field and allow a foothold for smaller players who do not have the capital or resources to train large models. Such companies can specialize in models for specific sectors and tasks or provide tools and services to organizations to create their own small models. "We will see small models everywhere," Goshen said. "We can already see them on the PC. We will see them in different configurations on various devices, and we will see very smart systems that will help choose which model to use and when."